AI saves me hours of time every week. But the single greatest ROI has been a GPT that took about an hour to build. (A 5000% return, and counting.)
GPTs allow anyone to create tailored versions of ChatGPT for specific tasks or domains. (Gems, recently released by Google, offer comparable functionality.)
It’s a way to train a Large Language Model on your custom instructions, then access that tool whenever you want.
But this isn’t a story about GPTs (though I will explain how to build one).
This is a story about scale.
Because as I’ve said: The secret about prompts is they sometimes take more time than the original task. It's not until they are used, repeatedly, that the real efficiencies are gained.
As a business owner, I use AI for many in-the-moment tasks: brainstorming, workshopping ideas, developing concepts.
This is for the other kind. The rote work, the mundane, the repetitive. The kind of work that, once delegated to AI, frees up you and your team to focus on more complex and strategic work.
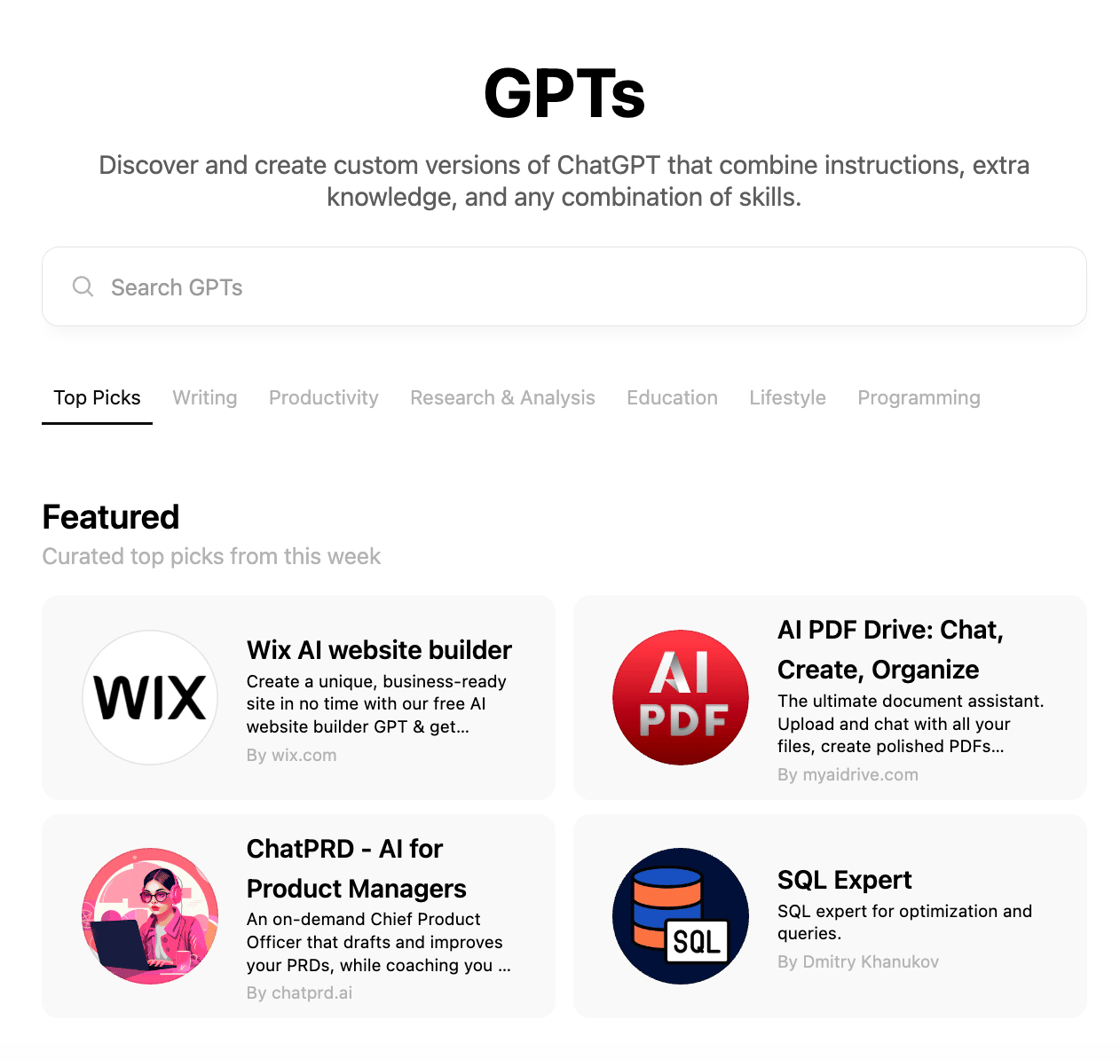
How we built a high-performing GPT (in about an hour)
When OpenAI introduced GPTs on November 6, 2023, we immediately saw the potential to streamline our client’s social media copywriting.
Here's how we did it, and what we learned along the way.
Our challenge: Real-time social media engagement
Our client's social media strategy revolved around reacting to and celebrating user-generated content — which required writing original, on-brand captions in almost real time. We knew we could increase our efficiency using AI.
Our approach: Building a custom GPT
1. Select the right use case
Not every task requires a custom GPT. Apply these criteria when considering a GPT:
- Repetitive: Is it something you do frequently?
- Structured: Do you have clear instructions, examples, or documented expertise?
- Narrow: Is the use case focused on a specific domain or task?
We evaluated our needs and realized that social media caption writing was an ideal candidate because it:
- Is an ongoing task (repetitive)
- Requires specific content types and brand voice (structured)
- Focuses solely on creating social media captions for one account (narrow)
We asked: Could a lengthy prompt do the job, or did we need the more advanced capabilities of a custom GPT?
We had hundreds of examples of sample captions, but it would have been cumbersome to attach these to every prompt for each new caption. This made a custom GPT the clear choice.
2. Gather relevant data
We knew that data would be essential. Fortunately, we already had:
- Brand and tone-of-voice standards.
- A documented social media strategy.
- Hundreds of published captions.
Of the prompting strategies tested, few-shot prompting (providing several examples) is one of the more successful.
By uploading a structured dataset (e.g. a clearly organized and labeled spreadsheet) of existing captions, we essentially built few-shot prompting into our GPT. This helped our GPT understand the specific patterns and styles we were aiming for.
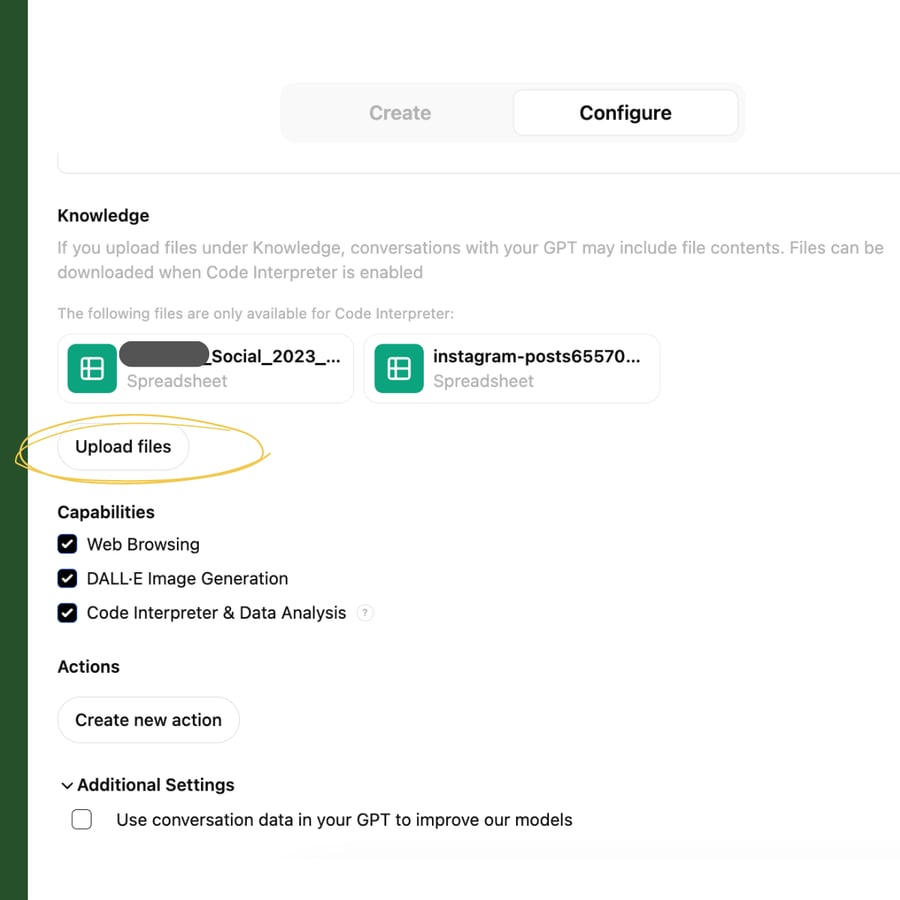
3. Craft clear instructions
We used our existing documentation to create instructions for our GPT:
- Assigned the GPT a role, which detailed the kinds of tasks it would perform.
- Outlined brand voice guidelines.
- Provided target audience information.
- Detailed content types and their specific requirements.
- Summarized do's and don'ts for brand communication.
If our spreadsheet of captions showed the final destination, the instructions explained how to get there.
4. Test, train, iterate
Creating our GPT was an iterative process. After our initial setup, we:
- Used sample prompts to test the GPT.
- Refined our instructions based on the results.
- Repeated the process until we achieved consistent, high-quality outputs.
This was not a lengthy process. Because of our commitment to keep a "human in the loop," testing could be done quickly and iteratively, knowing that we’d continue to maintain quality in our final product.
Once we'd added our instructions and uploaded files, our team could generate unlimited captions that mirrored the client's tone of voice, using prompts as simple as:
- Write a caption for this post.
- Give me 3 more examples.
- Here's the beginning of a caption; help me finish it.
- Write a funny response to the attached post.
5. Monitor and optimize over time
We've continued to improve our GPT's performance over time. We regularly:
- Update our instructions and dataset. Every few months, we upload more examples of published captions. This continues to reinforce the outputs we’d like to see, and the variety of forms that might take.
- Keep up with new features or capabilities of the underlying AI model. For example, once ChatGPT could reliably interpret text on images, we could provide screenshots of user-generated content instead of copying and pasting captions.

Our results one year later
Almost one year later, our custom GPT is still delivering impressive results and has even improved, thanks to our team's optimization and advancements in ChatGPT (improved multimodal capabilities, web browsing, etc.).
Here's what we've achieved:
- Task completion time reduced by approximately 50%.
- Maintained high-quality, brand-aligned content.
- Enabled exploration of creative, but still on-brand, ideas.
- Increased social media publishing frequency.
- Freed up time for more strategic content and campaign development.
The bottom line
We invested one hour to build a GPT, and we've saved one hour every single week since.
But this isn’t a story about GPTs. This is a story about scale.
And this story was made possible because we had already:
- Isolated a narrow, repetitive task. (Have you done this?)
- Documented our process and guidelines. (Do you have standard operating procedures? Are they in writing?)
- Identified exemplars. (What does ‘good’ look like?)
- Trained our team to be the “human in the loop.” (Can your doers become managers?)
This is one small story of how our workflows shift when we introduce AI. And how teams with documented systems and standards are better positioned to succeed.
There's another version of this story — one likely playing out in many businesses right now. With no plan, no process, and no guidelines, these companies end up with:
- Generic, mediocre outputs.
- Time lost to testing prompts and shifting approaches.
- Increasingly frustrated teams.
If adopting and scaling AI feels difficult, begin by looking at your processes: the documented steps, templates, guidelines, and standards that exist within your company. You might find that your problem does not lie with the technology, but with your systems.
You're reading a preview. Want more?
Tired of growth hacks and hucksters? Us, too.
So we created growthcurve: A newsletter for leaders in the thick of building companies.
Get practical frameworks, real-world stories, helpful templates, and nuanced advice to smooth out the bumps along the way.