“Never make the same mistake twice.” Navy SEALs conduct After-Action Reviews (AAR), a process to review and learn from both their mistakes and their accomplishments. They consider:
- What did we intend to accomplish?
- What happened?
- Why did it happen that way?
- What will we do next time?
Our first AI pilot project saved the client around $200,000 and hundreds of hours of work. But there were some big lessons learned, too. Let’s look at our top takeaways from this five-month project.
Backstory
Since 2021, we’ve been talking to our clients about AI — educating them on its uses and experimenting with it in small ways.
After more than a year of casual experimentation with AI, one client came to us with an ambitious goal: They wanted to test a new lead generation strategy (hyper-niche landing pages) and needed a lot of new content to support it. They wondered if we could apply AI to this project to decrease costs and increase speed to market.
It was spring 2023, ChatGPT’s GPT-4 model had just been released, and the timing felt right for a large-scale AI pilot.
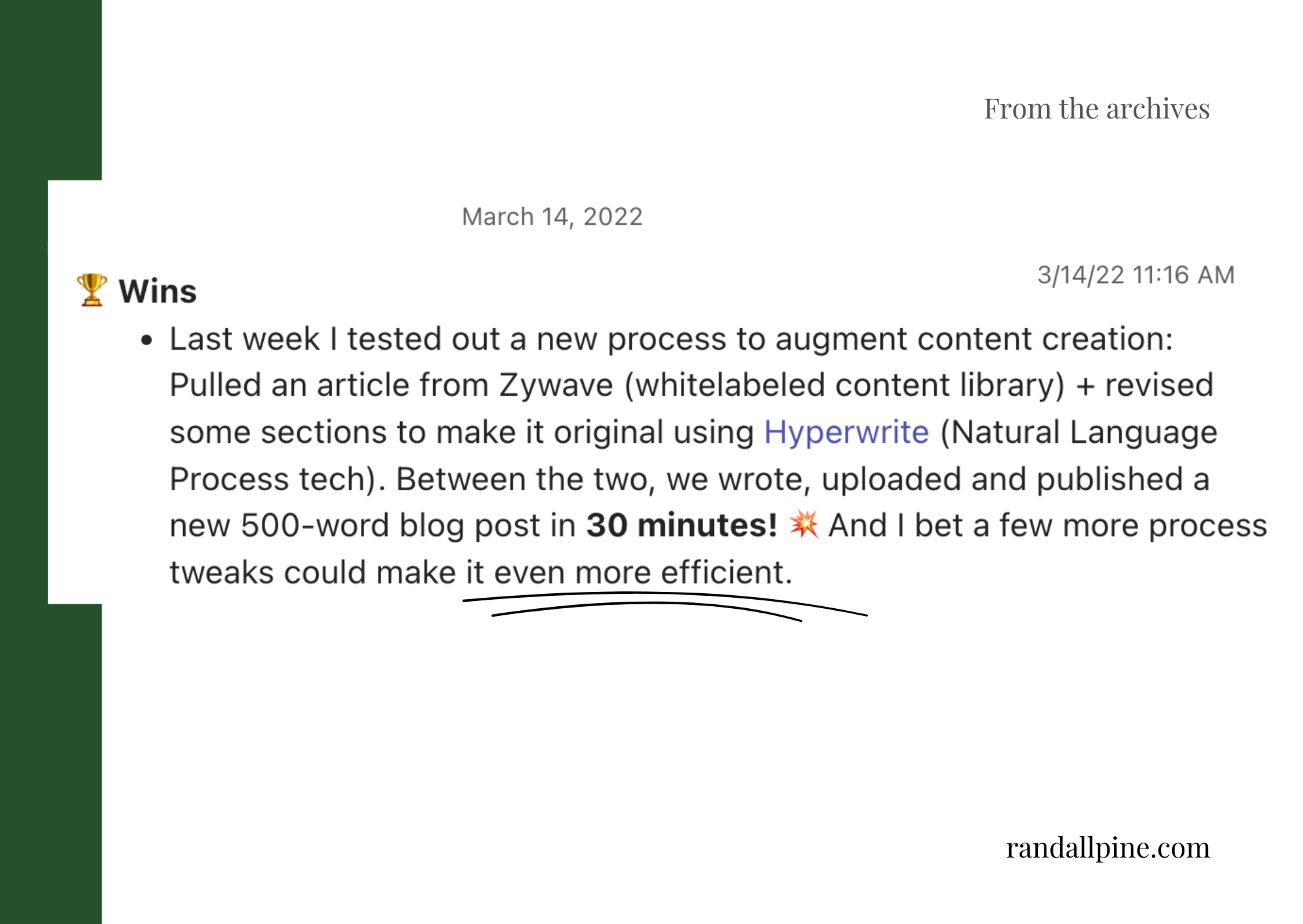
What We Did
The goal was to maximize efficiency and speed. Here’s what we did:
- Identified how we could use AI to speed up content creation, optimize pages for search engines, and format content. We then chose the best AI tools for each of these tasks.
- Developed clear, step-by-step guidelines for using AI, including how to input information into the chatbot, and how the team should review the AI-written content. The team had varying degrees of familiarity with AI, so every team member received clear instructions on which tools to use and how to use them, ensuring that all AI-generated content was thoroughly reviewed and edited by humans.
- Tested different ways to instruct and optimize AI to get the best results and minimize editing.
- Trained the marketing team and oversaw the project's rollout and execution.
- Started small. We began with about 15% of the total project to see how it went. Based on promising early results, we decided to move forward with the rest of the project.
- Continued to optimize as we scaled. Kept refining our methods and instructions to ensure we were getting the best quality content in the most efficient way.
What We Learned
3 Biggest Mistakes & Lessons Learned
Lesson #1: Don’t test a new strategy (if you can help it)
This project was an ideal use case for gen AI: Create a significant amount of content following a very similar outline. This meant it was highly scalable, and the initial investment in strategy and prompt engineering was worthwhile.
But it was also a new strategy the team was just testing out. Several, in fact:
- Hyper-targeted landing pages vs. appealing to broader buyer personas and broader, higher-volume keywords.
- Cross-selling several products at once.
- Targeting industry keywords (previously, their SEO strategy had been almost exclusively geo-targeted).
What I’d do differently next time: When piloting AI, your primary goals are to find a logical use case and get buy-in. But ideally, select a pilot project that would optimize an existing process. This way, the success of the project is evaluated solely on the impact of AI — not on the impact of AI and how well a new strategy performs.
Lesson #2: Experiment with prompting
Our earliest prompts were lengthy: A full page of instructions, attempting to prompt the LLM (large language model) to generate the entire web page in one shot.
Fortunately, we quickly learned that finding a "silver bullet" prompt would be more time-consuming (and still less reliable) than working iteratively. Instead, we broke prompts into smaller tasks, inserting human checkpoints for editing and fact-checking along the way.
We worked in stages:
- Conduct keyword research.
- Create an outline.
- Write the educational content sections.
- Write landing page copy for the call-to-action section.
- Write well-optimized subheadings.
- Add HTML tags to the final content.
This also helped set clear standards for the final product and the team's role in its delivery. (It was the early stages of what we now call Prompt Playbooks — a standard operating procedure built around a single AI prompt, or stringing together a series of prompts).
What I’d do differently next time: Looking back nearly one year later, today’s models may be able to generate the entire page from a single, well-written prompt. (Recently, I’ve been impressed with Gemini’s analytical capabilities and Claude Sonnet’s writing.) But still, given the chance to do it again, I would:
- Break the project up into smaller tasks.
- Create Prompt Playbooks for a handful of smaller, more specific prompts.
- Conduct early experiments with different prompts and different models, before settling on the top-performing ones.
- Consider creating the first page to provide as an example. (One-shot or few-shot prompting is, quite simply, giving AI an example and saying “do something like this.”)
Lesson #3: Content at scale = reviews at scale
We know gen AI can hallucinate, or generate inaccurate content, so we planned to have at least one team member review all copy.
Well, ChatGPT was so efficient that human reviews were slowing down the process. Once we fine-tuned our prompts, we were generating content at an incredible pace (even after factoring in human reviews, the project resulted in a 12x increase in hourly output). But that still pushed plenty of work downstream, where it had to be reviewed, uploaded, proofed, and published.
What I’d do differently next time: Set up our team with fewer writers and more editors and uploaders, to eliminate bottlenecks. And more broadly, consider how AI integration might impact existing roles and workflows.
3 Things We Did Well
Even with the mistakes made and lessons learned, we considered this pilot a huge win. Here are the top three things that, in hindsight, contributed to our success.
1. Use case selection
Setting aside the fact that it was a new strategy, the use case was a great candidate for AI: More than 100 pages that needed specific content, but with a significant amount of overlap, too.
This overlap in our content needs — combined with the large scale — meant that our initial investment in strategy, process, and prompting would pay off in the dozens and dozens of website pages that followed.
Gen AI also excels at processing tasks — like rewriting — because you’ve significantly narrowed its focus. (Remember one-shot prompting? That’s kind of what rewriting is: “Do something like this, just a bit different.”)
The takeaway: Be judicious with your use case selection — especially if it’s your company’s first experience with AI.
2. Organization
Creating that many pages required even more organization than I anticipated. (It’s amazing how dozens of pages can start to blur together.)
But our team created a solid plan that included:
- Templates: We had templates for just about everything — the page design, the content outline, the on-page content, the keyword optimization.
- Processes: We created a blueprint that we could easily follow as we scaled. Documented processes outlined which prompts we used and in what order, dictated file naming conventions and organization, and detailed how to review and proofread the final pages.
- Prompts: We used AI wherever we could, every step of the way — conduct keyword research, create optimized headlines, write a pillar page, localize this content, update this list of URLs, add HTML tags, and so on. All these prompts drove our process and later became the backbone of our prompt library.
The takeaway: Prioritize structure and organization during the planning phase. It’ll make execution that much easier and more efficient.
3. Data-driven approach
From the outset, our objective was clear: Create these pages in significantly less time than they would typically take, without sacrificing quality. Efficiency was the target.
As a marketing agency, we had years of time tracking data to benchmark our performance. This made it easy to set clear per-page targets and to calculate our increased efficiency and productivity afterward.
The takeaway: Consider your goal for each use case and how you can set your team up for success:
- Is it to improve efficiency? Then gather baseline data, and set up time tracking to calculate and compare.
- To increase productivity? Make a plan to track the team's pre-AI and post-AI output.
- To augment your team's skills? How do you plan to measure or demonstrate this increased ability? Document your approach.
Make sure your team is set up from the beginning to collect the right data.
If you want to learn more about how to select and run a successful AI pilot project, we created our newest service with you in mind. Get in touch with us to learn more.
Fast-track your company's responsible AI adoption with The 30-Day AI Ops Jumpstart.
Our team can help to help you:
- Pinpoint the best use cases for your company.
- Identify potential AI pilot projects.
- Standardize and centralize your top use cases in a prompt library.
- Develop a plan to put AI into action.